Recent Posts
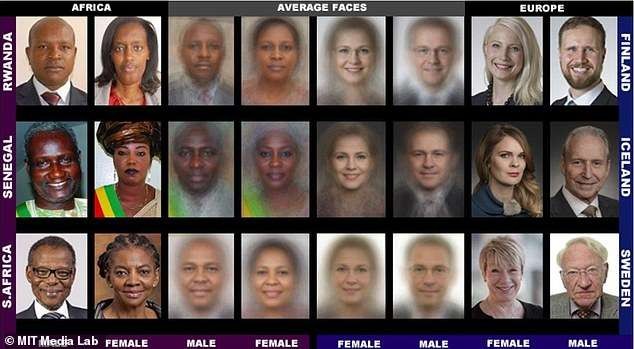
These past few weeks, I have been stationed at home in St Paul watching the protests in my proverbial backyard. George Floyd’s murder represents just another instance in which the power of the state systematically devalues Black life. As Ta-Nehisi Coates, in his book Between the World and Me, states “Here is what I would like for you to know: In America, it is traditional to destroy the black body—it is heritage.” Our entire political and legal system devalue Black life. Blacks are three times more likely to die from air pollution, Whites have ten-times the net-worth of black families most of this inequity due to the legacy of housing discrimination, even in healthcare Blacks are three-times more likely to die from Covid-19 than their white counterparts. This many not be intentional, but it is a legacy of how we consistently devalue black lives.
Black Americans for the past week have been protesting the fact that Floyd’s death, at the hands of a police officer, was one of many. They have been protesting the killing of Breonna Taylor; the killing of Ahmaud Arbery; the killings of Michael Brown and Philando Castile and Jamar Clark and Freddie Gray. They have been protesting state-sanctioned violence against black lives. They have been protesting police impunity. Their message is. clear. “Justice for George Floyd.” “Justice Can’t Wait.” “Black Lives Matter.” “I Can’t Breathe.”
But institutional violence and discrimination does not just exist in our criminal justice system. It pervades our entire socio-economic system. But as “software is eating the world”, it is leaving Americans of Color behind. Of greatest concern, however is the rise of autonomous vehicles and how tech’s unconscious bias against Black life will have deadly consequences.
In this post, I will discuss how AVs can disproportionately inflict violence on Black Americans and what we need to do to stop it.
AVs and AI ignore Black lives
In a seminal article, then-Stanford professor Lawrence Lessig directly attacked Seventh Circuit Judge Frank Easterbrook’s view that cyberspace law should be understood as merely applying old legal principles in new contexts. Not so, said Lessig, who asserted that society is constrained by different modalities, such as norms, markets, laws, and structural constraints. When the architecture of society changes, he posits people’s different responses make it difficult to apply traditional legal principles in these new contexts.
Lessig’s insight applies equally well to the problem of racial discrimination subsumed within the new technological setting of autonomous vehicles (AVs). The developers of AVs are not constrained by modalities that ensure that object-detection models recognize all skin colors at the same rate. (See Here). The rate at which current object detection systems in AVs show “false negatives” as to darker-skinned pedestrians—that is, fail to recognize them as pedestrians—is about 11 percent higher than the failure rate for lighter-skinned pedestrians.
As a federal appellate judge recently stated, discrimination, even when “shrouded in layers of legality is no less an insult to our Constitution than naked invidious discrimination.” As the current protest across the country show, we are not protesting just because of one mistake, but because of the systemic degradation of people of color intentional or not throughout our society.
Autonomous vehicles will be one the greatest technological shifts in the 21st century, but cannot implement this tech if it comes at the expense of the lives of one group of citizens.
Autonomous Vehicles Make Type-II Errors
Autonomous vehicles are some of the most advanced artificial intelligence systems being developed today. To make all the decisions needed to drive from one place to another without human input, autonomous vehicles adopt a “sense, plan, and act” process.” AVs use a variety of devices to perceive surrounding objects, develop a plan to navigate those objects, and then react to changes in the surrounding field as they put the plan into action. These processes are enormously complex. An AV not only has to perceive the static physical landscape, it needs to be aware of moving and non-moving objects such as pedestrians, other vehicles, cyclists, animals, and debris. It further has to operate on poorly marked roads and in adverse weather conditions.
Accordingly, most AVs rely on a suite of different sensors to recognize and classify the objects they encounter. Camera-based systems are the first line for AVs. All of these cameras rely on software systems to detect and classify objects. These camera systems are trained on datasets of millions of categorized images. The cars’ computer systems continue to learn to predictively recognize different objects based on previous examples. These cameras also need a high dynamic range (that is, the ability to perceive between the darkest and lightest objects) to deliver clear images even in direct sunlight or at night and to ensure that the cars get the most complete and accurate data to correctly recognize objects they encounter.
Yet, as recent accidents involving AVs have shown, these systems are still prone to recognition errors. Recent incidents include the death of Joshua Brown, who was killed when the Tesla he was in failed to recognize a truck that crossed his path, and an incident in Tempe, Arizona when an Uber AV hit a jaywalking pedestrian in 2018.
In 2016, Joshua Brown became the first American to die in an AV accident, when his Tesla Model S, operating in “autopilot” mode, failed to recognize an 18-wheeler. The 2015 Tesla had a forward radar system, a single forward-looking camera, and 12 long-range ultrasonic sensors. Afterwards, it was reported that the accident was likely directly related to the vehicle’s lack of additional sensors such as LIDAR.
The accident resulted from multiple recognition failures. As Brown traveled down a two-lane Florida highway, a tractor trailer made a left turn across traffic. Brown’s Tesla, traveling at highway speeds on autopilot, failed to recognize the truck. As the National Highway Traffic Safety Administration (NHTSA) reported, the camera failed to recognize the white truck against the brightly lit Florida sky. Further, the radar system failed to recognize the tractor trailer because it was trained to ignore objects such as signs, and mistook the truck for one of those “safe to ignore” objects.
Third, critics such as Jamie Condliffe, a writer for the MIT Technology Review, allege that a critical factor in the accident was Tesla’s refusal to use LIDAR in its mix of sensors. These critics note that unlike cameras, LIDAR works well in low light and glare and therefore provides more detailed and accurate data than radar or ultrasound. These critics conclude that if Tesla used a LIDAR system in its AVs, the cars would be much more likely to engage the brakes when an object appears in the car’s path. Some scholars argue this failure to use LIDAR breaches the duty of care.
Joshua Brown’s death was far from the only time that a Tesla has been involved in an automotive fatality while engaged in autopilot. Of six confirmed fatalities in accidents where vehicles were driving autonomously, five occurred in a Tesla. Furthermore, all accidents involving an AV where the driver died occurred in a Tesla.
However, another AV fatality—this time involving an Uber AV in Tempe, Arizona—suggests that even LIDAR sensors are no guarantee against recognition failures. In the 2018 accident, the Uber AV had a mix of sensors and cameras, including RADAR and LIDAR, but failed to identify biker Elaine Herzberg as she crossed the street outside of a crosswalk with her bicycle, until it was much too late to brake.[3] This incident sparked a significant outcry, leading critics such as Ethan Douglas, the head policy analyst at Consumer Reports, to call for putting “[s]mart, strong safety rules in place for self-driving vehicles,” noting that “there will always be companies that will skimp on safety” without adequate regulation.
AV Recognition Systems Fail to Recognize Darker Skin Tones
These type-II errors are endemic to the AV industry. But recent academic research indicates that recognition errors are more likely to affect darker-skinned Americans. In the study, researchers at Georgia State University tested state-of-the-art object-detection models currently used by AVs to determine whether rates of object recognition were affected by skin color. After controlling for time of day and occultation (when one object blocks another, causing sensors to fail to recognize it), researchers found that camera recognition models had an 11 percent accuracy gap in recognizing darker-skinned pedestrians. They also found that as they increased the precision (the number of true positives over actual results) on the training model, the number of false negatives for darker-skinned pedestrians rose, as compared to lighter-skinned pedestrians. Specifically, at the 75 average precision level, the researcher found that the models had an 11 percent statistical recognition gap. The researchers still went further and created additional subsets, because they wanted to exclude other factors such as time of day or occultation that might have affected the results. (Occultation is the process in which one object blocks another. In the context of AVs, this makes it more difficult for the AV to distinguish the two objects). They found that in each subset where they controlled for time of day and occultation, there was not a larger statistical difference between lighter-skinned and darker-skinned pedestrians. This led researchers to conclude these were not important factors that could explain the recognition gap.
Then, the researchers created a training data subset that had a heavier weighting of darker-skinned pedestrians. There, they found that the number of false negatives for darker-skinned pedestrians decreased by between 50 to 75 percent when they controlled for the weighting of the statistical sample. This research suggests that inequity in recognition likely stems from the weighting of the training data sets. This is a critical point: recognition failures are not necessarily caused by the technology, but by human decisions about how to train the technology. Those decisions can be addressed.
These accidents show AVs are still prone to Misidentifying objects and will Likely Misidentify Darker-Skinned People.
Autonomous vehicles that rely heavily on cameras (such as Teslas) have failed to recognize objects that have led to fatalities. The Uber AV accident also shows that no recognition system is fool-proof. The cause of type-II errors as it relates to issues around skin color seems is a flaw of improper weighting of training data sets—a number-crunching issue, not a software issue.
This problem of racial inequity in recognition systems is apparently driven by human decision making. Given the amount of injustice, people of color face this just strikes as another example of Silicon Valley hubris at the expense of marginalized society groups. By way of example, I will show how AVs left unchecked will lead to unacceptable deaths if left unchecked.
AVs and Race in the Year 2030
Imagine this hypothetical: In the year 2030, all vehicles are autonomous. Darker-skinned minorities represent 50 percent of the population. In that year, pedestrian deaths have dropped dramatically from 5,977 in 2017 (when deaths were split evenly among lighter- and darker-skinned populations) to 400, a near fourteen-fold decrease. Yet of those 400 fatalities, 300 are of dark-skinned minorities. In this example, all racial groups have benefited from a dramatically lower death rate, yet darker-skinned minorities are now significantly overrepresented among the remaining pedestrian fatalities.
From a purely economic perspective, this is an efficient, even desirable outcome. Pedestrian deaths have fallen dramatically, and economic loss has correspondingly decreased. Society is benefitted and enriched. But from a moral perspective, this is unacceptable. Permitting the use of technology that disproportionately killed dark-skinned pedestrians—even if it killed many fewer of them—would offend basic notions of equality That we have so often failed to provide in the past.
Silicon Valley Ignore Black Lives and What to do About it
As Professor Jennifer McAward argues, the constitution was devised in the post-Civil War era to create “…a system in which all people can engage in the basic transactions of civil life, regardless of race.”[1] Distributing causalities to darker-skinned minorities would offend the deeper principles of the post-Civil War and would be repugnant to Society.
Minorities in the United States suffer from the digital divide. Aproximately One in three African Americans and Hispanics — 14 million and 17 million, respectively — still don’t have access to computer technology in their homes. 35% of Black and 29% of Hispanic households do not have access to broadband. Meanwhile, Black and Latinos represent such a disproportionately low percentage of Silicon Valley’s workforce the U.S. Equal Employment Opportunity Commission used the language “segregated” to define the current situation.
Silicon Valley’s anthem has always been “move fast and break things,” but that cannot be the case when thing that it breaks are people’s lives. AVs have the ability to inflict disparate violence as a consequence of “algorithmic oversight.” While this type of discrimination is of the type subjected to George Floyd it, nonetheless, still fits the narrative in which society continues to devalue Black life. Technology has great promise for the future and I for one welcome Autonomous Vehicles ability to increase road safety. Yet I for one, thinks it would be morally repugnant if we allow these vehicles on the road and use minorities’ deaths to work out the bugs.
Senator Booker has introduced legislation that would hold companies liable for algorithmic bias in their corporate policies. In order to address this issue, Congress needs to hold automakers accountable for unintentional algorithmic bias in the same manner. Please contact your legislator and demand Algorithmic Accountability from automakers.